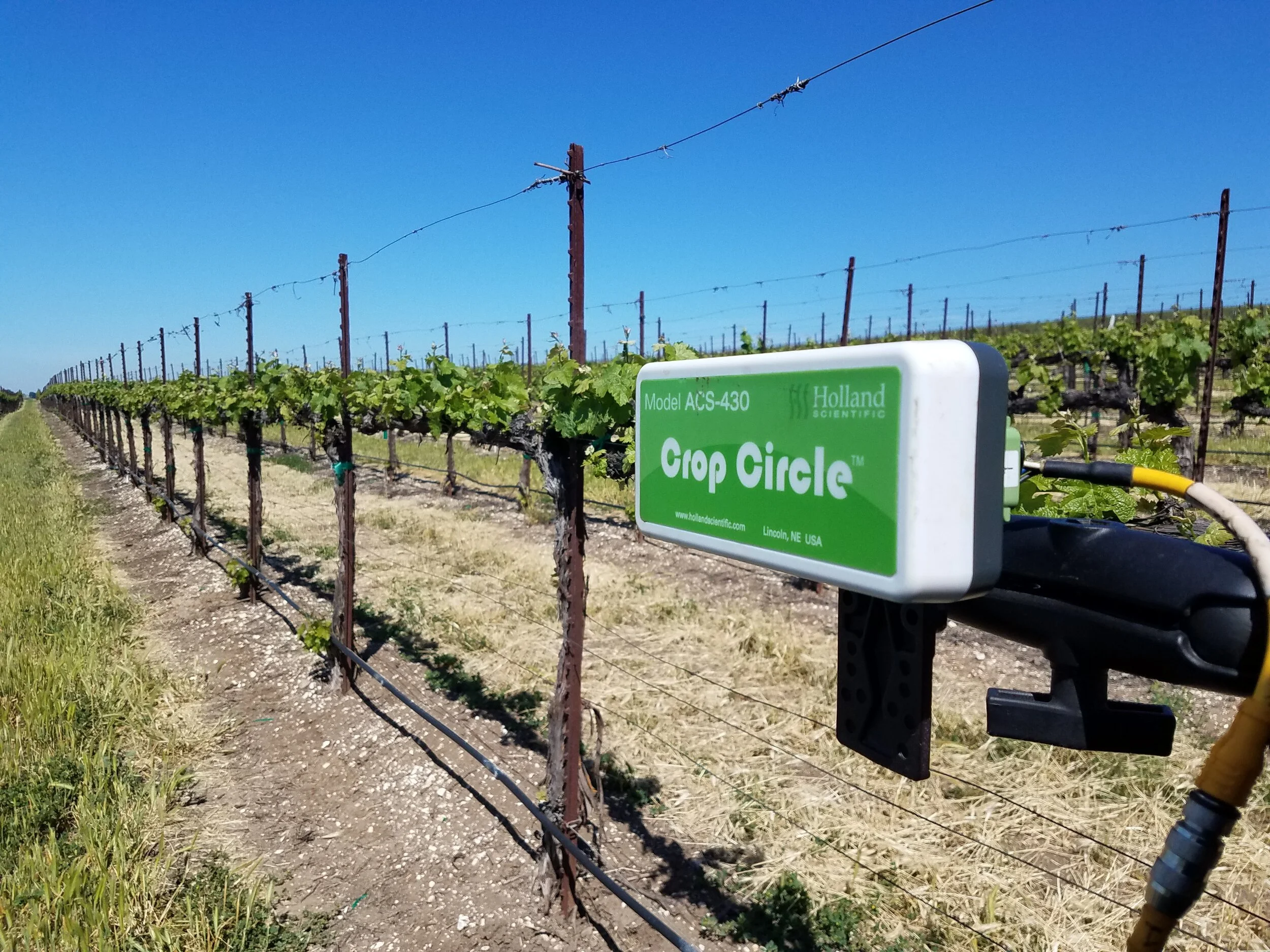
Integrating Technology and Viticulture Research for High Quality Grape Production
Funding for the Efficient Vineyard Project comes from the USDA-NIFA-Specialty Crop Research Initiative competitive grants program and our industry sponsors
Precision Vineyard Management: Collecting and Interpreting Spatial Data for Variable Vineyard Management
SCRI Project Number: 2015-51181-24393
The Efficient Vineyard Approach
Measure
Use agricultural sensors to collect data on vineyard soils, canopy development, crop size, and fruit quality.
Model
Integrate sensor data and directed field measurements into useful viticulture information for vineyard managers
Manage
Develop variable-rate vineyard prescription maps to improve yield and quality, lower production costs, and conserve labor and environmental resources.
Project History
2005
Old school viticulture…new technology
Traditional vineyard soil and vine measurements, such as soil moisture and vine pruning weight, are geo-referenced and mapped with developing GPS technology.
2006-2010
Exploring proximal sensors in vineyards
Following global efforts in precision viticulture, Dr. Terry Bates at Cornell begins investigating off-the-shelf sensors, grape yield monitor technology, and spatial data mapping in NY Concord vineyards. Spatial variation in commercial vineyards proves to be high, supporting the future potential in using sensor-GPS technology to map and manage vineyard productivity.
2011
Cornell Viticulture connects with Carnegie Mellon Engineering
Steve Nuske from Carnegie Mellon University develops prototype imaging system to non-destructively count fruit and map yield in Cornell research vineyards.
2012
NGRA funds pilot study
National Grape and Wine Initiative (precursor to NGRA) funds pilot study on spatial vineyard crop load mapping. Precision Agriculture scientist, James Taylor, joins the Cornell Lake Erie Research team and elevates the level of spatial data processing and interpretation.
2013
Multi-layered mapping for vine balance
Vine balance is an integrated relationship between soil properties, canopy growth, and crop size. Soil (DualEM), Canopy (CropCircle NDVI), Crop (CMU image unit), and yield (ATV yield monitor) sensors are used to demonstrate the complexity within and between spatial vineyard layers.
2014
Real vineyards…real applications
As part of the NGRA pilot study, soil, canopy, and crop sensors are deployed in juice, wine, and table grape vineyards to understand the degree and pattern of spatial variation under commercial conditions.
To address the need for non-destructive crop imaging under field conditions, Carnegie Mellon develops the first field rugged version of the grapevine image system.
2015
USDA Funds the Efficient Vineyard SCRI Project
The SCRI Project, Precision Vineyard Management: Collecting and Interpreting Spatial Data for Variable Vineyard Management, is funded by the USDA. Activities move from sensor exploration and validation to generating information for precision vineyard management.
2016
Efficient Vineyard Phase 1: Improving Measurement Tools for the PV Toolbox
Validation of commercial sensors: Soil, canopy, and crop sensors are validated against manual in-field measurements to translate spatial sensor data into vineyard information, such as canopy fill and vine pruning weight.
Non-destructive berry detection: George Kantor’s engineering team further develops the CMU vineyard image system to non-destructively detect berry number and berry diameter. This is turned into a visible berry count and spatially mapped to visualize crop distribution and improve vineyard crop estimation.
Multi-layer spatial-data processing: Raw data from all of the sensors are processed by a technique which allows comparison between information layers.
Does the soil pattern influence vine growth? Where and how much? Is fruit sugar dominated by yield or vine balance in this vineyard? Can I address this through variable rate management? Multi-layer processing helps answer these types of questions.
2017
Efficient Vineyard Phase 2: Modeling Viticulture and Spatial Data
Distilling layers into management maps: Ultimately, all this data collection needs to be turned into smart management decisions. Different methods of generating spatial prescription maps are tested under commercial conditions.
Stratified vineyard sampling based on spatial data: Sensor data are relatively inexpensive and spatially dense but an indirect measure of soil, canopy, or crop. Manual vineyard sampling is expensive and sparse but measures what the vineyard manager needs. Putting the two together is a powerful tool in generating meaningful vineyard maps.
Viticulture modeling of vine balance: Crop load, an indicator of vine balance, is the relationship between vine yield and pruning weight. Using EV techniques, spatial maps of yield and vine size are used to generate vineyard crop load maps to determine levels of vine stress and fruit quality.
2018
Efficient Vineyard Phase 3: Turning information into variable-rate management
Predicting color development in table grape vineyards: The CMU image system successfully tracks spatial color development in CA vineyards and predicts percent harvestable fruit for the next week, influencing harvest and labor decisions.
Spatial-data driven variable-rate vineyard mechanization: The EV project integrates spatial prescription maps with precision agriculture software and vineyard mechanization implements to deliver variable-rate applications. Successful variable-rate mechanization both reduces vineyard labor costs and improves overall vineyard balance.
On-the-fly Yield and Juice soluble solids: Evaluation of commercial yield monitors and the development of a Brix monitor allows simultaneous mapping during harvest operations, important for evaluating past management and planning for the next season.
2019
Efficient Vineyard Phase 4: Tools for the Industry
Dissemination of project information: The EV website, project webinars, field demonstrations, seminars, and the Nelson J. Shaulis symposium in Digital Agriculture provide educational materials for stakeholders.
Technology Commercialization: The CMU imaging hardware and software technology was successfully moved to Bloomfield AI for further commercial development in the grape industry.
The MyEfficientVineyard tool for growers: We found that one of the major barriers to adoption for small to medium size grape growers is in having access to spatial data processing. In cooperation with Orbitist, we have developed a web-based tool to upload, process, and visualize sensor data.